Table of Contents
ToggleThe Hidden Cost of Intelligent Machines
Artificial intelligence may be an invisible force in our daily lives, but it has a very real physical footprint. Every ChatGPT query, image generation, or algorithmic recommendation is powered by electricity-hungry data centers. And as AI adoption explodes, so does its demand for energy – raising urgent questions about whether our climate goals can withstand this new digital drain on the power grid.
Consider this: training a single state-of-the-art AI model can consume astonishing amounts of electricity. OpenAI’s GPT-4 model, for example, required an estimated 42.4 gigawatt-hours (GWh) of electricity for its training – roughly equivalent to the daily electricity use of 28,500 U.S. homes. That’s just to teach the model. Once deployed, these AI models draw power every time they respond to a prompt. In fact, the energy usage from running popular generative AI tools at scale is already immense. One analysis by the International Energy Agency in 2024 found that the electricity consumed by ChatGPT queries over a year exceeded the annual power used for all Google searches worldwide by about 10 terawatt-hours. These eye-opening numbers drive home a simple truth: today’s AI isn’t just smart – it’s power-hungry.
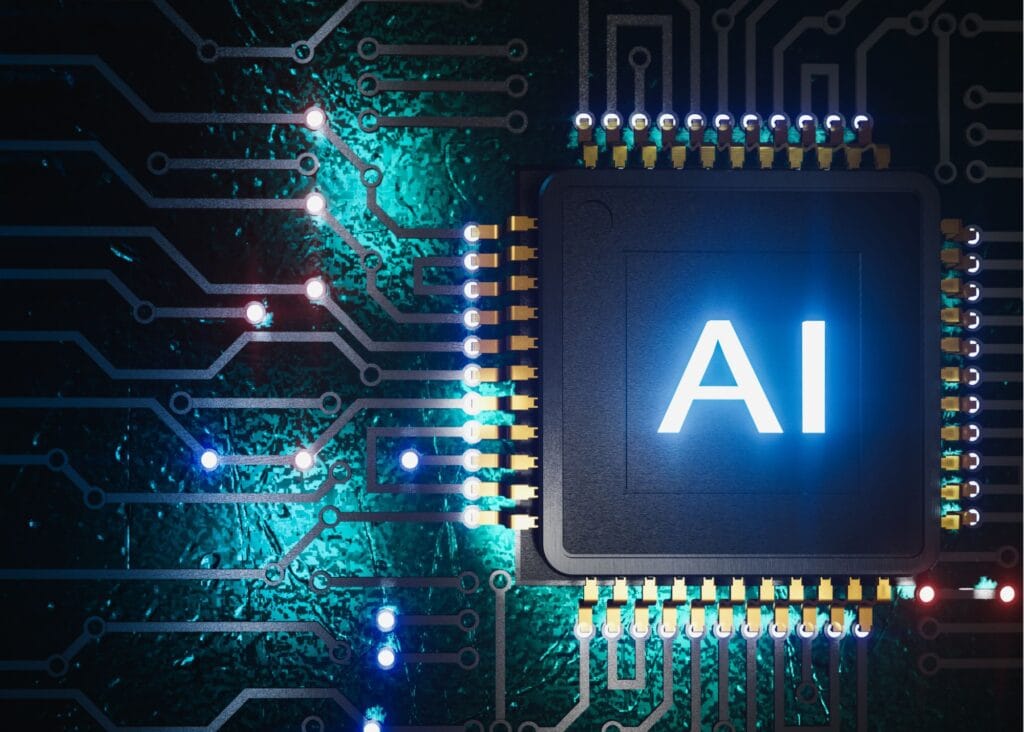
Much of that hunger comes from the sheer computational intensity of modern AI. Training large language models means running trillions of operations on specialized hardware for days or weeks on end. Even after training, serving millions of users with AI-generated text or images (the inference stage) demands around-the-clock computing on power-thirsty graphics processing units (GPUs) in the cloud. “AI servers use up to 10 times the power of a standard server, and companies are deploying them at an unprecedented scale,” notes Eric Masanet, a sustainability scientist who studies data center energy use. In other words, an AI-optimized server farm can draw tenfold more electricity than a conventional data center of similar size – and right now tech companies are racing to build as many as they can. The combination of high-power hardware and rapid expansion is straining the grid, Masanet warns.
This surge is recent and rapid. Industry experts describe the current moment as the “AI acceleration” era – an explosion in data center energy demand driven by AI workloads. Since late 2022, when generative AI went mainstream, hyperscale cloud providers and startups alike have been scrambling to deploy larger models and serve a growing user base. The result: a new wave of data center construction and hardware upgrades around the world, all to accommodate AI’s needs. Each new server warehouse that comes online brings thousands of additional processors devoted to AI – and a proportionate jump in electricity consumption.
Data Centers: America’s New Power Hogs
If AI is the brain of our modern economy, then data centers are its beating heart – and that heart is drawing unprecedented power. Nowhere is this more evident than in the United States, which is experiencing a data center building boom. Northern Virginia, the world’s largest data center hub, offers a striking example: the total floor space of server farms in that region now spans the equivalent of eight Empire State Buildings filled with computers. And each building-sized data center can consume as much electricity as a mid-sized town.
Nationwide, analysts say we are witnessing something historic. “For the first time in decades, America needs to produce more electricity” to meet rising demand, observes a recent report from a state energy committee. Unlike past growth spurts driven by new factories or population booms, today’s uptick is largely due to clusters of power-hungry data centers. These facilities have become so energy-intensive that they are poised to overtake heavy industry in electricity consumption. By the end of this decade, the U.S. is on track to use more electricity for running data centers than it does for producing aluminum, steel, cement, chemicals and all other energy-intensive products combined. In advanced economies overall, data centers could account for over 20% of all growth in power demand through 2030 – reversing a trend of stagnating electricity usage and putting new pressure on power grids.
Globally, the numbers are equally sobering. The International Energy Agency (IEA) released a major report in April highlighting AI’s outsized role in driving demand. It projects that worldwide electricity use by data centers will more than double by 2030 to around 945 terawatt-hours (TWh) – slightly more than the entire annual consumption of Japan. And that may be a conservative scenario. A separate analysis by the International Monetary Fund estimates that AI’s rapid uptake could more than triple data center electricity needs to roughly 1,500 TWh by 2030 – about as much power as India consumes today. For context, an increase of that magnitude within a single decade would mean data centers jump from consuming ~1% of global electricity to consuming well over 3%.
Such growth has caught even energy experts off guard. “AI is one of the biggest stories in the energy world today – but until now, policy makers and markets lacked the tools to fully understand the wide-ranging impacts,” said Fatih Birol, the IEA’s Executive Director, upon releasing the new findings. The impacts are indeed wide-ranging. In the United States, data centers (driven by AI usage) are forecast to account for almost half of the growth in U.S. electricity demand through 2030. In power-hungry emerging AI markets like Japan and Malaysia, data centers could make up an outsized share of new demand as well. This puts policymakers in a bind: how to supply all this electricity without derailing climate commitments.
The immediate response from industry has been a construction blitz. Tech giants are pouring billions into new server farms. Google, for instance, expects to invest a record $75 billion in capital expenditures in 2025, largely on data centers, servers and network gear to expand its AI cloud capacity. Microsoft and Amazon are following suit, racing to build out infrastructure for AI services. But adding so many massive facilities so quickly is upending local energy planning. In some regions, big data center projects have raised alarms about grid reliability and fairness. Legislators in states like New Jersey and South Carolina worry that ordinary customers could see higher electric bills as utilities upgrade infrastructure to feed energy-hungry AI farms. “We have a crisis coming our way in electric rates. These outrageous increases are going to be put on the citizens. Why should they bear the rate increases?” asks New Jersey State Senator Bob Smith, who has proposed requiring new AI data centers in his state to secure their own clean power supply so that the public isn’t stuck with the tab. More than a dozen similar proposals are now percolating in state legislatures across the country, aiming to ensure the AI revolution doesn’t unwittingly saddle consumers with rising energy costs.
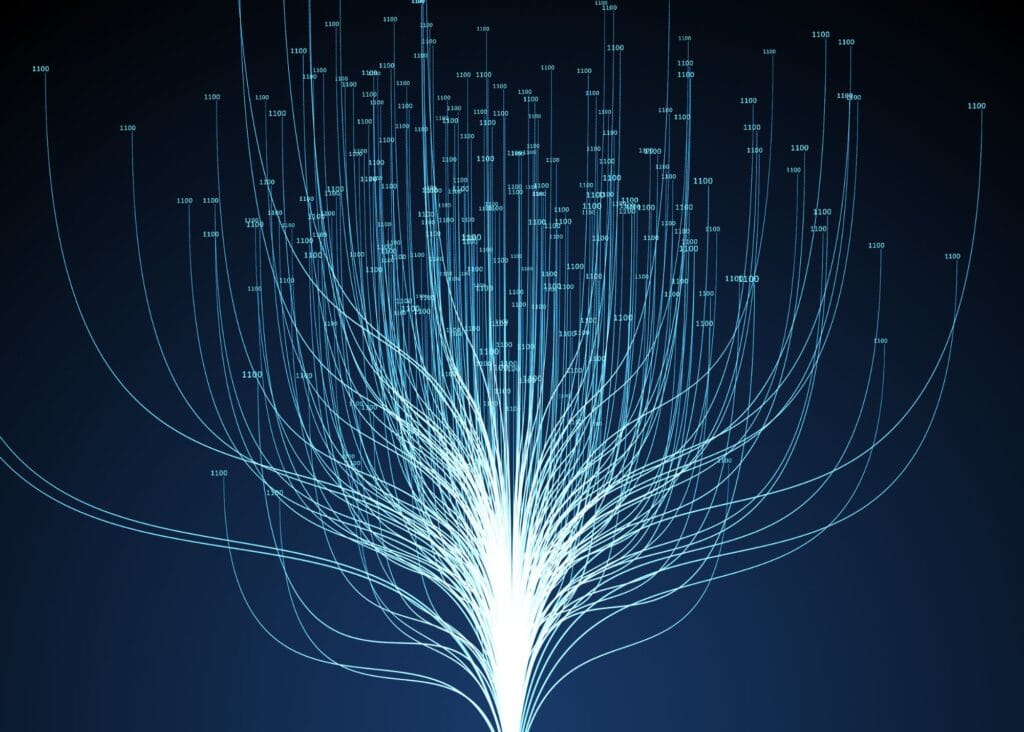
The Climate Risks of Unchecked AI Growth
What does this surging energy demand mean for the climate? In simple terms, if left unchecked, AI’s growth could seriously undermine efforts to cut greenhouse emissions – but the scale of that impact will depend on how we manage the energy supply. Right now, much of the electricity fueling our AI boom comes from fossil fuels. In the U.S., for example, natural gas power plants currently provide the bulk of electricity for data centers, and are expected to shoulder most of the increased load through 2030. In China, the second-largest market for AI, data centers are predominantly coal-powered today. Unless the power mix changes, a doubling or tripling of data center energy use means a similar explosion in carbon emissions. The IEA warns that, on a business-as-usual path, emissions from the electricity used by data centers could rise by as much as 180% by 2035 – making it one of the fastest-growing sources of carbon pollution. And that figure doesn’t even count the “embodied” emissions from manufacturing millions of new servers, pouring concrete for new buildings, or producing the steel and semiconductors that an AI-enabled digital economy requires.
To appreciate the stakes, consider some concrete examples of AI’s environmental footprint. Researchers at UC Riverside and Caltech recently found that training a single advanced model (Meta’s LLaMA-3.1 in their study) produced as much air pollution in the form of particulate emissions as 10,000 car trips from Los Angeles to New York and back. This pollution largely comes from the diesel backup generators and coal plants that kick in to support energy-intensive training runs. The public health costs aren’t trivial – the same study estimated $190–$260 million in annual health damages from the increased air pollution due to data centers running AI models. Meanwhile, running those giant server farms also guzzles water: Data centers use water for cooling, often millions of gallons per day for a single facility. Pulling that much water from local supplies – and later discharging warmed water – can exacerbate drought conditions and even raise the risk of wildfires in arid regions. In the desert city of Phoenix, Arizona, which is rapidly becoming an AI data center hotspot, officials worry that growth is outpacing the area’s water limits and electrical grid capacity.
Perhaps the most direct threat to climate goals is the possibility that booming AI demand could prop up fossil fuel infrastructure just when we need to phase it out. This scenario is already starting to play out. In Northern Virginia – that data center epicenter – utilities have had to keep aging coal and gas plants online to meet the surging load from server farms, even as those plants were slated for retirement. In a striking recent development, the U.S. administration indicated interest in leveraging coal power specifically for AI: an executive order signed in April seeks to identify regions where idle coal-fired plants could be repurposed to supply new AI data centers. While proponents argue this ensures energy “security” for AI growth, it flies in the face of decarbonization efforts. Funneling more electricity from coal – the dirtiest fuel – to feed AI would make it far harder for the U.S. to meet its emissions targets. In effect, AI could become an unwitting driver of a fossil fuel resurgence, locking in high-carbon energy sources at the precise moment the world is trying to ditch them.
Not everyone is convinced that AI’s rise will spell climate doom. The International Monetary Fund’s analysis found that, even accounting for the extra energy use, AI’s net impact might be economically positive. They project AI could add about 0.5 percentage points to annual global GDP growth between now and 2030 – and that the social cost of the related emissions (roughly $50–66 billion by their estimate) is minor compared to the economic gains. Moreover, some experts argue that AI, if applied wisely, could help drive down emissions in other sectors – for example, by optimizing energy use in industries, improving power grid efficiency, or accelerating research into climate-friendly technologies. A study by the Grantham Institute on Climate Change suggests AI could even lead to an overall reduction in emissions if it speeds up breakthroughs in clean power, transportation, or agriculture. It’s the idea that AI might become a powerful tool in humanity’s climate toolkit, offsetting its own footprint by enabling larger carbon savings elsewhere.
However, realizing those climate benefits from AI is far from guaranteed. Roberta Pierfederici, a policy fellow at the Grantham Institute, cautions that “market forces alone are unlikely to successfully drive AI’s application toward climate action.” In her view, governments, tech companies and energy providers must actively steer AI to be used “intentionally, equitably and sustainably”, rather than assuming it will naturally align with climate goals. In other words, without deliberate efforts and policies, the default trajectory of AI is more likely to overwhelm power systems than to revolutionize them for good. There’s also a fundamental challenge in the AI research community itself: a relentless drive toward ever-larger models and more complex algorithms. This “bigger is better” mentality has delivered impressive AI capabilities, but at an environmental cost. As sustainability researcher Alex de Vries points out, this dynamic makes AI fundamentally incompatible with long-term environmental sustainability unless something changes. Each new generation of AI model often requires an order-of-magnitude more data and computation, setting up an exponential growth curve in resource consumption. Any hope of limiting AI’s power draw, de Vries argues, depends on breaking this cycle or hitting external constraints – for instance, if physical grid capacity or costs simply can’t keep up.
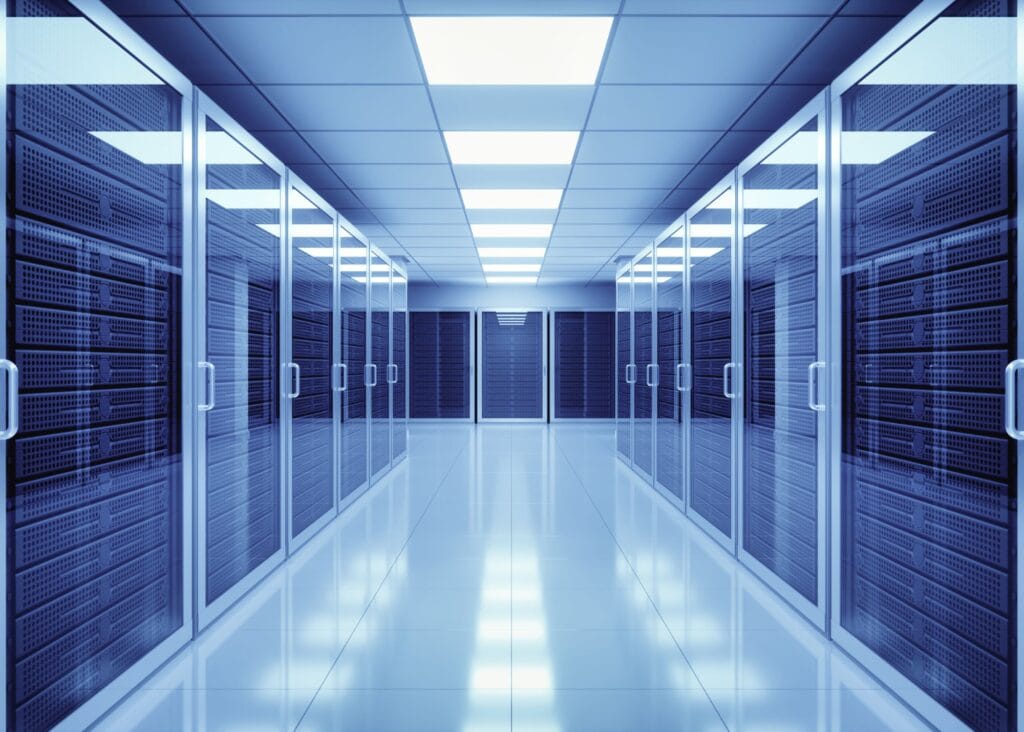
Can Innovation Make AI Greener?
The good news is that awareness of AI’s energy problem is growing, and a variety of innovations and strategies are emerging to blunt its environmental impact. Tech companies, policymakers, and scientists are all scrambling for solutions – from better chips to smarter code to cleaner power sources. Here are some of the promising approaches under discussion to mitigate AI’s footprint:
- Clean Energy Integration: Perhaps the most straightforward solution is to power AI with renewable energy instead of fossil fuels. Major cloud providers have announced ambitious targets to run their data centers on 100% carbon-free power. For example, Google has signed on to the Climate Neutral Data Centre Pact in Europe, committing to net-zero emissions operations by 2030. Microsoft, Amazon, and others are investing heavily in wind and solar farms and buying renewable energy certificates to match their AI electricity use. In fact, Amazon is now the world’s largest corporate purchaser of renewable energy, part of an effort to ensure its expanding cloud (including AI services) is as green as possible. However, as Alex de Vries has noted, even Google is struggling to source enough clean energy to keep up with skyrocketing demand. The reality today is that AI expansion is outpacing the rollout of renewables in many regions. Some companies are eyeing unconventional options to secure carbon-free power: Microsoft has even considered reopening a nuclear power plant – the retired Three Mile Island reactor in Pennsylvania – to provide emissions-free electricity for its AI data centers. And a coalition of tech firms (including Google, Meta and Amazon) is backing efforts to scale up small modular nuclear reactors as a steady clean power source for future data centers. The push for clean energy is encouraging, but scaling it fast enough to meet AI’s voracious appetite remains a monumental challenge.
- Efficient Hardware and Software: AI engineers are also racing to make each computation more efficient, so that we get more intelligence out of every watt of power. There has been progress on this front. According to the Stanford 2025 AI Index, AI-specific hardware is becoming dramatically more efficient – improving energy efficiency by around 40% per year on average, even as costs drop. Think of custom AI chips like Google’s Tensor Processing Units (TPUs) or NVIDIA’s latest GPUs that can do more operations per joule of energy than previous generations. Similarly, datacenter designers have gotten better at squeezing efficiency out of cooling and electrical systems. Modern hyperscale data centers are far more efficient than the fragmented server rooms of a decade ago, often operating with a power usage effectiveness (PUE) close to 1.1 (meaning very little overhead energy is wasted on cooling or other needs). These gains have helped temper the growth in energy use – but not reverse it. There’s a constant tug-of-war between efficiency improvements and the rebound effect, where gains just enable even larger models and more usage. For instance, a new algorithmic technique might cut the compute needed per AI inference by 50%, but if it allows the model to be used by twice as many people or to incorporate 10× more data, total energy use may still rise. The IEA’s special report flagged this rebound effect: even AI models marketed as “efficient” can end up consuming more total electricity if they scale to millions of users. Still, pursuing efficiency is crucial. Researchers are exploring techniques like “mixture-of-experts” architectures that activate only portions of a model as needed, radically reducing computations for each task. One such model, DeepSeek, claimed to match its larger competitors’ performance with much lower training energy by using this approach – though an analysis found its inference usage still ended up quite high in practice. Algorithmic optimizations (like better training routines, model compression, and more efficient software libraries) can also chip away at energy waste. Every incremental gain – from smarter chips to streamlined code – helps bend the curve.
- Geographic and Temporal Optimization: Another strategy is moving AI workloads to places or times when clean energy is abundant. Unlike a factory tied to a specific location, AI computation can be somewhat flexible about where it runs. Companies are exploring geographical load shifting – for example, running power-intensive AI jobs in data centers located in regions with lots of hydro, wind, or solar power (such as Iceland or the Pacific Northwest). Likewise, temporal shifting can play a role: non-urgent AI tasks might be scheduled for midday when solar farms are producing at peak, or overnight when wind power might otherwise go unused. This kind of smart scheduling ensures that AI is using the greenest electrons available at any given moment. Some cloud providers have tools to dynamically route workloads based on real-time grid carbon intensity. These approaches effectively make AI chase the wind and sun, reducing reliance on fossil-fueled electricity.
- Cooling and Infrastructure Innovations: Data center operators are also innovating to reduce auxiliary energy use. Since nearly 40% of a data center’s energy can go into cooling the servers, improving cooling efficiency has a big impact. Techniques like advanced liquid cooling (circulating coolant directly to hot chips) can cut down the electricity needed for cooling compared to traditional air conditioning. Some operators are even experimenting with submerging servers in special fluids or situating data centers underwater, taking advantage of natural heat dissipation. Other infrastructure tweaks – from better airflow management to heat recycling – are being employed to squeeze more useful work out of each kilowatt that enters a facility.
- Policy and Regulation: Finally, policymakers are starting to step in with standards and incentives to align AI’s growth with climate goals. On the regulatory side, there are proposals for energy efficiency standards for large data centers, requirements for transparency in reporting AI-related energy use and emissions, and even moratoria or limits on new data centers in regions with constrained grids. In Europe, discussions are underway about treating major cloud data centers as part of critical infrastructure that must meet certain green criteria (building on voluntary agreements like the Climate Neutral Data Centre Pact). At the U.S. state level, as noted, some lawmakers want to condition data center tax breaks on using renewable power or to cap the subsidies if a project would otherwise drive up local energy prices. These moves aim to ensure that companies internalize the cost of providing clean power for their AI growth rather than externalizing it to society. There’s also a role for public investment: government R&D programs can support development of energy-efficient AI algorithms and electrical grid upgrades, while infrastructure legislation can accelerate the build-out of renewables and transmission lines needed to accommodate data center loads. “Countries that want to benefit from AI need to quickly accelerate investments in electricity generation and grids, improve data center efficiency, and strengthen dialogue between policy makers, the tech sector and the energy industry,” advises the IEA in its recent report. In short, a coordinated effort is needed to prevent AI’s power demands from overwhelming decarbonization efforts.
Even with all these initiatives, experts caution that there is no silver bullet. “Even with efficiency gains, AI’s energy footprint is still expected to grow,” Masanet told UCSB’s The Current. “The key is ensuring that this growth aligns with sustainable energy deployment rather than exacerbating fossil fuel dependence.” In other words, we likely can’t stop AI’s energy demand from increasing in the near term – but we can choose whether that increase comes from clean sources or dirty ones, and whether we moderate it through efficiency or let it run rampant. Masanet is moderately optimistic that today’s exponential surge will level off a bit: some AI business models may prove unsustainable, and data centers won’t run at full tilt 24/7 if demand saturates. But planning for a high-demand future is prudent.
A Delicate Balance for the Future
As we sprint into an AI-driven future, we stand at a crossroads: will artificial intelligence be a help or hindrance in the fight against climate change? On one hand, AI offers unprecedented tools to optimize systems and drive innovation in clean energy – potentially a powerful ally in reducing emissions. On the other hand, the way we’re building and deploying AI today is creating an energy sink of formidable proportions, one that could put climate targets further out of reach. Whether AI becomes an asset or a liability for the climate largely depends on choices we make now.
The challenge is to reconcile AI’s insatiable appetite for power with the planet’s finite carbon budget. That means urgently expanding renewable energy capacity, investing in grid resilience, and making sustainability a core criterion in AI development. It also means having honest conversations about trade-offs. Do we really need ever-bigger models for every task, or can the next breakthroughs focus on doing more with less? Can the tech sector’s competitive “AI arms race” pivot to a race for the most energy-efficient algorithms? These questions demand input not just from CEOs and engineers, but from regulators, scientists, and the public.
Encouragingly, there is growing recognition within the AI community of the need for responsibility. Leading AI conferences now host workshops on “green AI”, and some organizations are exploring standards for reporting the carbon footprint of AI research. This cultural shift, combined with the pressure of climate reality, might instill a new norm: that progress in AI should be measured not only in accuracy or speed, but also in efficiency and sustainability. After all, an AI model that dazzles with its intelligence but exacts an unacceptable toll on the environment is a bad trade for humanity in the long run.
As we mark this year’s Earth Day, the juxtaposition of two trends – blistering advances in AI and mounting urgency of climate action – is a reminder that technological progress and sustainability must walk hand in hand. There is no pause button on innovation, but there is an opportunity to guide it. If we succeed in greening AI’s power supply and curbing its waste, we may look back on this period as one where two great challenges of our time found a harmonious path forward. If we fail, we could see our climate goals overshadowed by a digital behemoth we created.
The stakes are high, but so is the motivation to solve this puzzle. As the IEA’s Fatih Birol put it, AI is a tool – an incredibly powerful one – but it’s up to us how we use it. The same technology that threatens to guzzle gigawatts could also help us manage energy smarter and accelerate clean energy breakthroughs. Our task now is to ensure that the AI revolution is powered by clean innovation, so that the pursuit of machine intelligence doesn’t unintentionally sabotage the fight against climate change. In the end, the true measure of “intelligence” for this industry may be how wisely it manages its own footprint on our fragile planet.